CELLxGENE: scRNA-seq¶
CZ CELLxGENE hosts the globally largest standardized collection of scRNA-seq datasets.
LaminDB makes it easy to query the CELLxGENE data and integrate it with in-house data of any kind (omics, phenotypes, pdfs, notebooks, ML models, …).
You can use the CELLxGENE data in two ways:
Query collections of
AnnData
objects (this page).Query a big array store produced by concatenated
AnnData
objects viatiledbsoma
(see here).
If you are interested in building similar data assets in-house:
See the transfer guide to zero-copy data to your own LaminDB instance.
See the scRNA guide for how to create a growing versioned queryable scRNA-seq dataset.
See the Curate for validating, curating and registering your own AnnData objects.
Show me a screenshot
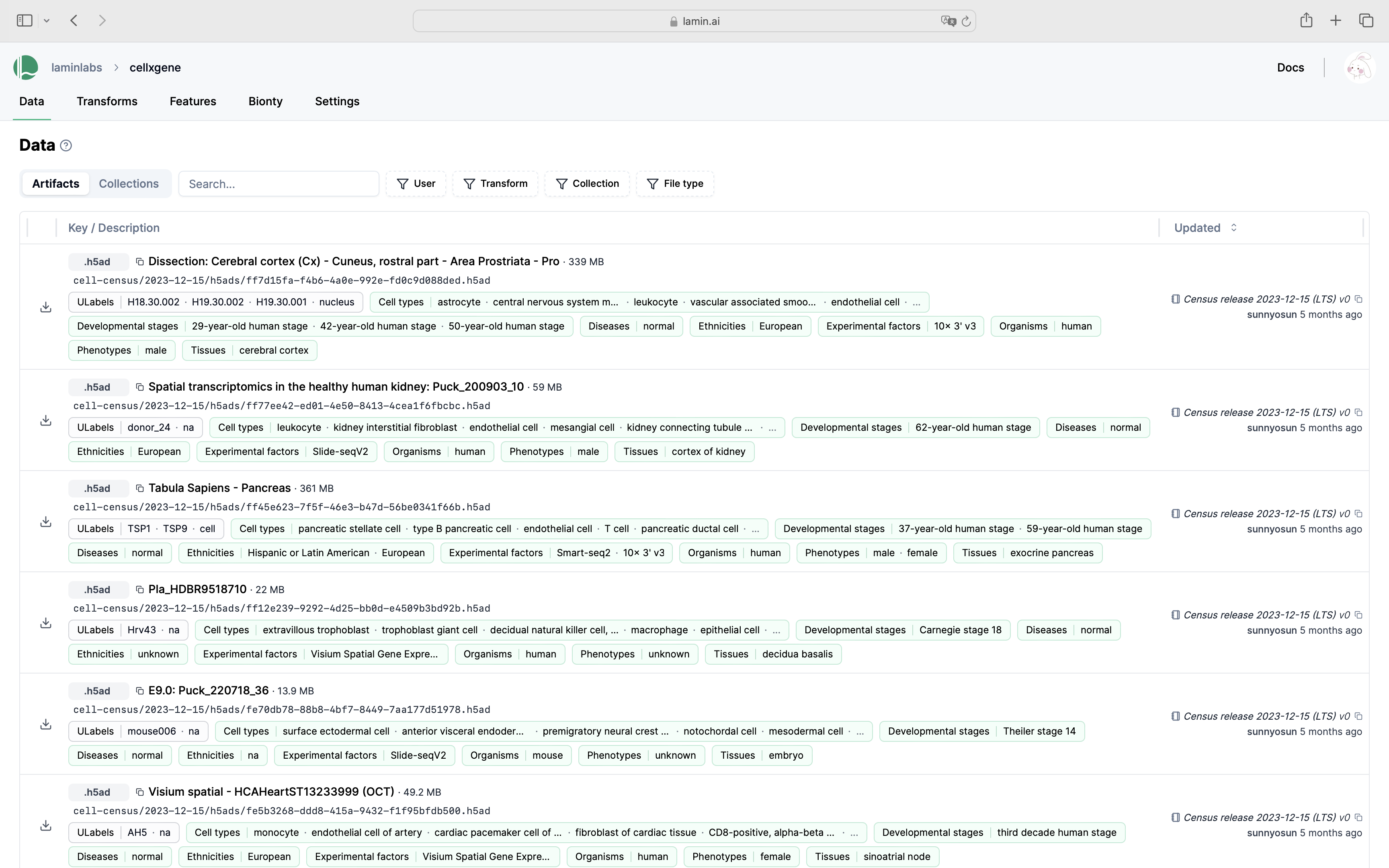
Load the public LaminDB instance that mirrors cellxgene:
# !pip install 'lamindb[bionty,jupyter]'
!lamin load laminlabs/cellxgene
❗ Full backed capabilities are not available for this version of anndata, please install anndata>=0.9.1.
💡 connected lamindb: laminlabs/cellxgene
import lamindb as ln
import bionty as bt
Show code cell output
💡 connected lamindb: laminlabs/cellxgene
❗ Full backed capabilities are not available for this version of anndata, please install anndata>=0.9.1.
Query & understand metadata¶
Auto-complete metadata¶
You can create look-up objects for any registry in LaminDB, including basic biological entities and things like users or storage locations.
Let’s use auto-complete to look up cell types:
Show me a screenshot
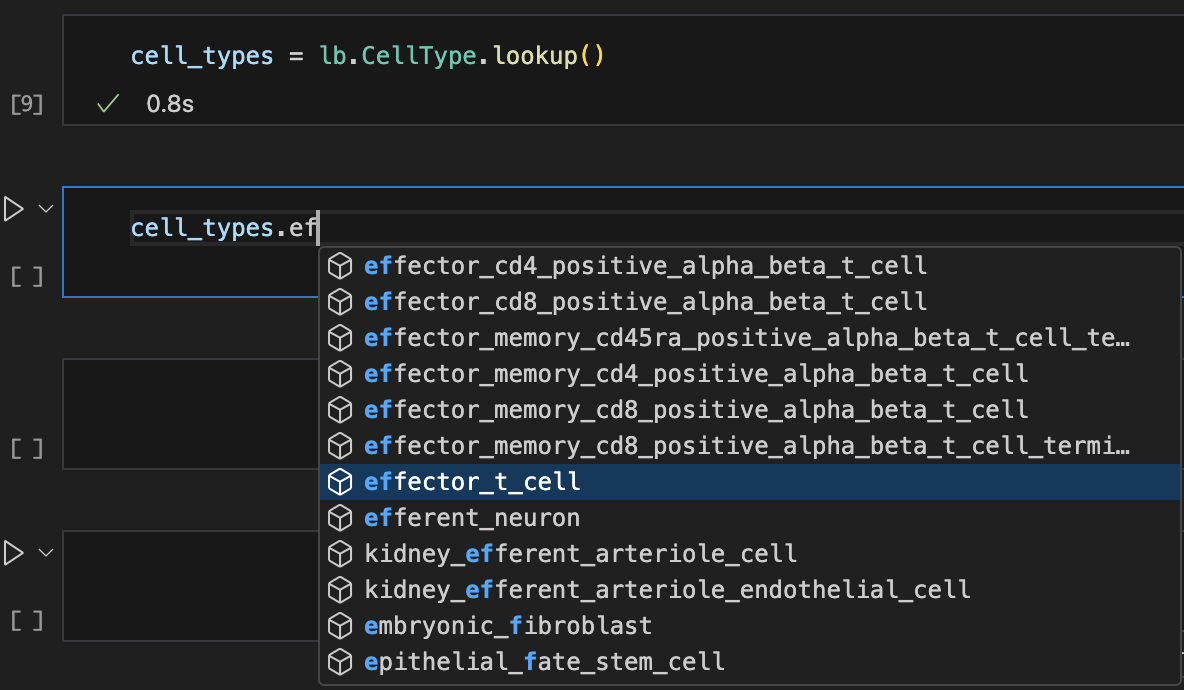
cell_types = bt.CellType.lookup()
cell_types.effector_t_cell
CellType(uid='3nfZTVV4', name='effector T cell', ontology_id='CL:0000911', synonyms='effector T-cell|effector T-lymphocyte|effector T lymphocyte', description='A Differentiated T Cell With Ability To Traffic To Peripheral Tissues And Is Capable Of Mounting A Specific Immune Response.', created_by_id=1, source_id=48, updated_at='2023-11-28 22:30:57 UTC')
You can also arbitrarily chain filters and create lookups from them:
users = ln.User.lookup()
organisms = bt.Organism.lookup()
experimental_factors = bt.ExperimentalFactor.lookup() # labels for experimental factors
tissues = bt.Tissue.lookup() # tissue labels
suspension_types = ln.ULabel.filter(name="is_suspension_type").one().children.lookup() # suspension types
Search & filter metadata¶
We can use search & filters for metadata:
bt.CellType.search("effector T cell").df().head()
Show code cell output
uid | name | ontology_id | abbr | synonyms | description | source_id | run_id | created_by_id | updated_at | |
---|---|---|---|---|---|---|---|---|---|---|
id | ||||||||||
1623 | 3nfZTVV4 | effector T cell | CL:0000911 | None | effector T-cell|effector T-lymphocyte|effector... | A Differentiated T Cell With Ability To Traffi... | 48 | NaN | 1 | 2023-11-28 22:30:57.481778+00:00 |
1503 | 1oa5G2Mq | memory T cell | CL:0000813 | None | memory T-cell|memory T lymphocyte|memory T-lym... | A Long-Lived, Antigen-Experienced T Cell That ... | 48 | NaN | 1 | 2023-11-28 22:27:55.580290+00:00 |
1169 | 6JD5JCZC | CD8-positive, alpha-beta cytokine secreting ef... | CL:0000908 | None | CD8-positive, alpha-beta cytokine secreting ef... | A Cd8-Positive, Alpha-Beta T Cell With The Phe... | 48 | NaN | 1 | 2023-11-28 22:27:55.571576+00:00 |
1229 | 69TEBGqb | exhausted T cell | CL:0011025 | None | Tex cell|An effector T cell that displays impa... | None | 48 | NaN | 1 | 2023-11-28 22:27:55.572884+00:00 |
1331 | 43cBCa7s | helper T cell | CL:0000912 | None | helper T-lymphocyte|T-helper cell|helper T lym... | A Effector T Cell That Provides Help In The Fo... | 48 | NaN | 1 | 2023-11-28 22:27:55.575955+00:00 |
And use a uid
to filter exactly one metadata record:
effector_t_cell = bt.CellType.filter(uid="3nfZTVV4").one()
effector_t_cell
CellType(uid='3nfZTVV4', name='effector T cell', ontology_id='CL:0000911', synonyms='effector T-cell|effector T-lymphocyte|effector T lymphocyte', description='A Differentiated T Cell With Ability To Traffic To Peripheral Tissues And Is Capable Of Mounting A Specific Immune Response.', created_by_id=1, source_id=48, updated_at='2023-11-28 22:30:57 UTC')
Understand ontologies¶
View the related ontology terms:
effector_t_cell.view_parents(distance=2, with_children=True)
Or access them programmatically:
effector_t_cell.children.df()
uid | name | ontology_id | abbr | synonyms | description | source_id | run_id | created_by_id | updated_at | |
---|---|---|---|---|---|---|---|---|---|---|
id | ||||||||||
931 | 2VQirdSp | effector CD8-positive, alpha-beta T cell | CL:0001050 | None | effector CD8-positive, alpha-beta T lymphocyte... | A Cd8-Positive, Alpha-Beta T Cell With The Phe... | 48 | None | 1 | 2023-11-28 22:27:55.565981+00:00 |
1088 | 490Xhb24 | effector CD4-positive, alpha-beta T cell | CL:0001044 | None | effector CD4-positive, alpha-beta T lymphocyte... | A Cd4-Positive, Alpha-Beta T Cell With The Phe... | 48 | None | 1 | 2023-11-28 22:27:55.569832+00:00 |
1229 | 69TEBGqb | exhausted T cell | CL:0011025 | None | Tex cell|An effector T cell that displays impa... | None | 48 | None | 1 | 2023-11-28 22:27:55.572884+00:00 |
1309 | 5s4gCMdn | cytotoxic T cell | CL:0000910 | None | cytotoxic T lymphocyte|cytotoxic T-lymphocyte|... | A Mature T Cell That Differentiated And Acquir... | 48 | None | 1 | 2023-11-28 22:27:55.575444+00:00 |
1331 | 43cBCa7s | helper T cell | CL:0000912 | None | helper T-lymphocyte|T-helper cell|helper T lym... | A Effector T Cell That Provides Help In The Fo... | 48 | None | 1 | 2023-11-28 22:27:55.575955+00:00 |
Query artifacts¶
Unlike in the SOMA guide, here, we’ll query sets of .h5ad
files, which correspond to AnnData
objects.
ln.Artifact.filter(
suffix=".h5ad",
description__contains="immune",
size__gt=1000000000,
cell_types__name__in=["B cell", "T cell"],
created_by__handle="sunnyosun"
).order_by(
"created_at"
).df(
include=["cell_types__name", "created_by__handle"]
).head()
cell_types__name | created_by__handle | uid | version | description | key | suffix | type | accessor | size | ... | hash_type | n_objects | n_observations | visibility | key_is_virtual | storage_id | transform_id | run_id | created_by_id | updated_at | |
---|---|---|---|---|---|---|---|---|---|---|---|---|---|---|---|---|---|---|---|---|---|
879 | [conventional dendritic cell, classical monocy... | sunnyosun | BCutg5cxmqLmy2Z5SS8J | 2023-07-25 | Type I interferon autoantibodies are associate... | cell-census/2023-07-25/h5ads/01ad3cd7-3929-465... | .h5ad | None | AnnData | 6353682597 | ... | md5-n | None | 600929 | 1 | False | 2 | 11 | 16 | 1 | 2024-01-24 07:14:10.959155+00:00 |
1106 | [immature B cell, monocyte, naive thymus-deriv... | sunnyosun | 3xdOASXuAxxJtSchJO3D | 2023-07-25 | HSC/immune cells (all hematopoietic-derived ce... | cell-census/2023-07-25/h5ads/48101fa2-1a63-451... | .h5ad | None | AnnData | 6214230662 | ... | md5-n | None | 589390 | 1 | False | 2 | 11 | 16 | 1 | 2024-01-24 07:11:10.324135+00:00 |
1174 | [monocyte, conventional dendritic cell, plasma... | sunnyosun | wt7eD72sTzwL3rfYaZr2 | 2023-07-25 | A scRNA-seq atlas of immune cells at the CNS b... | cell-census/2023-07-25/h5ads/58b01044-c5e5-4b0... | .h5ad | None | AnnData | 1052158249 | ... | md5-n | None | 130908 | 1 | False | 2 | 11 | 16 | 1 | 2024-01-24 07:09:45.364255+00:00 |
1377 | [monocyte, ciliated cell, macrophage, natural ... | sunnyosun | znTBqWgfYgFlLjdQ6Ba7 | 2023-07-25 | Large-scale single-cell analysis reveals criti... | cell-census/2023-07-25/h5ads/9dbab10c-118d-496... | .h5ad | None | AnnData | 13929140098 | ... | md5-n | None | 1462702 | 1 | False | 2 | 11 | 16 | 1 | 2024-01-24 07:14:24.084706+00:00 |
1482 | [effector CD4-positive, alpha-beta T cell, con... | sunnyosun | dEP0dZ8UxLgwnkLjz6Iq | 2023-07-25 | Single-cell sequencing links multiregional imm... | cell-census/2023-07-25/h5ads/bd65a70f-b274-413... | .h5ad | None | AnnData | 1204103287 | ... | md5-n | None | 167283 | 1 | False | 2 | 11 | 16 | 1 | 2024-01-24 07:05:49.602044+00:00 |
5 rows × 21 columns
Queries by string are easily full of typos. Let’s query with auto-completed records instead:
ln.Artifact.filter(
suffix=".h5ad",
description__contains="immune",
size__gt=1000000000,
cell_types__in=[cell_types.b_cell, cell_types.t_cell],
created_by=users.sunnyosun
).order_by(
"created_at"
).df(
include=["cell_types__name", "created_by__handle"]
).head()
cell_types__name | created_by__handle | uid | version | description | key | suffix | type | accessor | size | ... | hash_type | n_objects | n_observations | visibility | key_is_virtual | storage_id | transform_id | run_id | created_by_id | updated_at | |
---|---|---|---|---|---|---|---|---|---|---|---|---|---|---|---|---|---|---|---|---|---|
879 | [conventional dendritic cell, classical monocy... | sunnyosun | BCutg5cxmqLmy2Z5SS8J | 2023-07-25 | Type I interferon autoantibodies are associate... | cell-census/2023-07-25/h5ads/01ad3cd7-3929-465... | .h5ad | None | AnnData | 6353682597 | ... | md5-n | None | 600929 | 1 | False | 2 | 11 | 16 | 1 | 2024-01-24 07:14:10.959155+00:00 |
1106 | [immature B cell, monocyte, naive thymus-deriv... | sunnyosun | 3xdOASXuAxxJtSchJO3D | 2023-07-25 | HSC/immune cells (all hematopoietic-derived ce... | cell-census/2023-07-25/h5ads/48101fa2-1a63-451... | .h5ad | None | AnnData | 6214230662 | ... | md5-n | None | 589390 | 1 | False | 2 | 11 | 16 | 1 | 2024-01-24 07:11:10.324135+00:00 |
1174 | [monocyte, conventional dendritic cell, plasma... | sunnyosun | wt7eD72sTzwL3rfYaZr2 | 2023-07-25 | A scRNA-seq atlas of immune cells at the CNS b... | cell-census/2023-07-25/h5ads/58b01044-c5e5-4b0... | .h5ad | None | AnnData | 1052158249 | ... | md5-n | None | 130908 | 1 | False | 2 | 11 | 16 | 1 | 2024-01-24 07:09:45.364255+00:00 |
1377 | [monocyte, ciliated cell, macrophage, natural ... | sunnyosun | znTBqWgfYgFlLjdQ6Ba7 | 2023-07-25 | Large-scale single-cell analysis reveals criti... | cell-census/2023-07-25/h5ads/9dbab10c-118d-496... | .h5ad | None | AnnData | 13929140098 | ... | md5-n | None | 1462702 | 1 | False | 2 | 11 | 16 | 1 | 2024-01-24 07:14:24.084706+00:00 |
1482 | [effector CD4-positive, alpha-beta T cell, con... | sunnyosun | dEP0dZ8UxLgwnkLjz6Iq | 2023-07-25 | Single-cell sequencing links multiregional imm... | cell-census/2023-07-25/h5ads/bd65a70f-b274-413... | .h5ad | None | AnnData | 1204103287 | ... | md5-n | None | 167283 | 1 | False | 2 | 11 | 16 | 1 | 2024-01-24 07:05:49.602044+00:00 |
5 rows × 21 columns
Query artifacts via collections¶
To access them, we query the Collection
record that links the latest LTS set of .h5ad
artifacts:
collection = ln.Collection.filter(name="cellxgene-census", version="2024-07-01").one()
collection
Collection(uid='dMyEX3NTfKOEYXyMKDD7', version='2024-07-01', name='cellxgene-census', hash='nI8Ag-HANeOpZOz-8CSn', visibility=1, created_by_id=1, transform_id=22, run_id=27, updated_at='2024-07-16 12:24:38 UTC')
You can get all linked artifacts as a dataframe - there are >1000 h5ad files in cellxgene-census
version 2023-12-15
.
collection.artifacts.count()
812
collection.artifacts.df().head() # not tracking run & transform because read-only instance
Show code cell output
uid | version | description | key | suffix | type | accessor | size | hash | hash_type | n_objects | n_observations | visibility | key_is_virtual | storage_id | transform_id | run_id | created_by_id | updated_at | |
---|---|---|---|---|---|---|---|---|---|---|---|---|---|---|---|---|---|---|---|
id | |||||||||||||||||||
3305 | 1BNWhcCqu1CMSJaHxpbn | 2024-07-01 | All - A single-cell transcriptomic atlas chara... | cell-census/2024-07-01/h5ads/98e5ea9f-16d6-47e... | .h5ad | dataset | AnnData | 2578203515 | k-aZJBIjuvnO5Vek3JK-Mg-308 | md5-n | None | 110824 | 1 | False | 2 | 22 | 27 | 1 | 2024-07-12 12:40:41.719518+00:00 |
3301 | aJTH55LW2CTIWu306YiY | 2024-07-01 | Supercluster: Deep-layer intratelencephalic | cell-census/2024-07-01/h5ads/98113e7e-f586-406... | .h5ad | dataset | AnnData | 3521994530 | B8cjeVHgg9Q9Rr-JGaUjfg-420 | md5-n | None | 228467 | 1 | False | 2 | 22 | 27 | 1 | 2024-07-12 12:40:41.728651+00:00 |
3313 | pnQX4jvkj3eFWGOzDxbW | 2024-07-01 | Evolution of cellular diversity in primary mot... | cell-census/2024-07-01/h5ads/9b686bb6-1427-4e1... | .h5ad | dataset | AnnData | 107509355 | Z-uGNA6tRhMB1q46A3R8yg-13 | md5-n | None | 10739 | 1 | False | 2 | 22 | 27 | 1 | 2024-07-12 12:40:41.762869+00:00 |
3566 | 2bF2gDSwbNbDsFVg2KQf | 2024-07-01 | Supercluster: CGE-derived interneurons | cell-census/2024-07-01/h5ads/e4ddac12-f48f-445... | .h5ad | dataset | AnnData | 2586217727 | 8IDdkinp07n9AgQaWH9yUw-309 | md5-n | None | 129495 | 1 | False | 2 | 22 | 27 | 1 | 2024-07-12 12:40:42.069642+00:00 |
2879 | Pvhx7GAmAt4SYg03sE0M | 2024-07-01 | Single nucleus transcriptomic profiling of hum... | cell-census/2024-07-01/h5ads/06ef6b36-6c9b-4e1... | .h5ad | dataset | AnnData | 92790726 | V9KkecqXGqQJRF1lluo6Kg-12 | md5-n | None | 10533 | 1 | False | 2 | 22 | 27 | 1 | 2024-07-12 12:34:51.739962+00:00 |
You can query across artifacts by arbitrary metadata combinations, for instance:
query = collection.artifacts.filter(
organisms=organisms.human,
cell_types__in=[cell_types.dendritic_cell, cell_types.neutrophil],
tissues=tissues.kidney,
ulabels=suspension_types.cell,
experimental_factors=experimental_factors.ln_10x_3_v2,
)
query = query.order_by("size") # order by size
query.df().head() # convert to DataFrame
Show code cell output
uid | version | description | key | suffix | type | accessor | size | hash | hash_type | n_objects | n_observations | visibility | key_is_virtual | storage_id | transform_id | run_id | created_by_id | updated_at | |
---|---|---|---|---|---|---|---|---|---|---|---|---|---|---|---|---|---|---|---|
id | |||||||||||||||||||
2961 | WwmBIhBNLTlRcSoBDt76 | 2024-07-01 | Mature kidney dataset: immune | cell-census/2024-07-01/h5ads/20d87640-4be8-487... | .h5ad | dataset | AnnData | 45158726 | GCMHkdQSTeXxRVF7gMZFIA-6 | md5-n | None | 7803 | 1 | False | 2 | 22 | 27 | 1 | 2024-07-12 12:40:43.756335+00:00 |
2961 | WwmBIhBNLTlRcSoBDt76 | 2024-07-01 | Mature kidney dataset: immune | cell-census/2024-07-01/h5ads/20d87640-4be8-487... | .h5ad | dataset | AnnData | 45158726 | GCMHkdQSTeXxRVF7gMZFIA-6 | md5-n | None | 7803 | 1 | False | 2 | 22 | 27 | 1 | 2024-07-12 12:40:43.756335+00:00 |
3000 | gHlQ5Muwu3G9pvFCx3x8 | 2024-07-01 | Fetal kidney dataset: immune | cell-census/2024-07-01/h5ads/2d31c0ca-0233-41c... | .h5ad | dataset | AnnData | 64546349 | 2qy8uy-65Sd_XcBU-nrPgA-8 | md5-n | None | 6847 | 1 | False | 2 | 22 | 27 | 1 | 2024-07-12 12:40:45.273783+00:00 |
3324 | P4Oai3OLGAzRwoicHfLM | 2024-07-01 | Mature kidney dataset: full | cell-census/2024-07-01/h5ads/9ea768a2-87ab-46b... | .h5ad | dataset | AnnData | 194047623 | aZVpGZwAfMCziff_5ow2bg-24 | md5-n | None | 40268 | 1 | False | 2 | 22 | 27 | 1 | 2024-07-12 12:40:44.478948+00:00 |
3324 | P4Oai3OLGAzRwoicHfLM | 2024-07-01 | Mature kidney dataset: full | cell-census/2024-07-01/h5ads/9ea768a2-87ab-46b... | .h5ad | dataset | AnnData | 194047623 | aZVpGZwAfMCziff_5ow2bg-24 | md5-n | None | 40268 | 1 | False | 2 | 22 | 27 | 1 | 2024-07-12 12:40:44.478948+00:00 |
Query arrays¶
Each artifact stores an array in form of an curated data matrix, an AnnData
object.
Let’s look at the first array in the artifact query and show metadata using .describe()
:
artifact = query.first()
artifact.describe()
Show code cell output
Artifact(uid='WwmBIhBNLTlRcSoBDt76', version='2024-07-01', description='Mature kidney dataset: immune', key='cell-census/2024-07-01/h5ads/20d87640-4be8-487f-93d4-dce38378d00f.h5ad', suffix='.h5ad', type='dataset', accessor='AnnData', size=45158726, hash='GCMHkdQSTeXxRVF7gMZFIA-6', hash_type='md5-n', n_observations=7803, visibility=1, key_is_virtual=False, updated_at='2024-07-12 12:40:43 UTC')
Provenance
.created_by = 'sunnyosun'
.storage = 's3://cellxgene-data-public'
.transform = 'Census release 2024-07-01 (LTS)'
.run = '2024-07-16 12:49:41 UTC'
Labels
.organisms = 'human'
.tissues = 'cortex of kidney', 'renal medulla', 'kidney', 'kidney blood vessel', 'renal pelvis'
.cell_types = 'classical monocyte', 'plasmacytoid dendritic cell', 'natural killer cell', 'dendritic cell', 'CD4-positive, alpha-beta T cell', 'mast cell', 'neutrophil', 'non-classical monocyte', 'CD8-positive, alpha-beta T cell', 'B cell', ...
.diseases = 'normal'
.phenotypes = 'male', 'female'
.experimental_factors = '10x 3' v2'
.developmental_stages = '2-year-old human stage', '4-year-old human stage', '12-year-old human stage', '44-year-old human stage', '49-year-old human stage', '53-year-old human stage', '63-year-old human stage', '64-year-old human stage', '67-year-old human stage', '70-year-old human stage', ...
.ethnicities = 'unknown'
.ulabels = 'TxK2', 'Wilms1', 'TxK4', 'TTx', 'RCC3', 'RCC1', 'VHL', 'TxK3', 'TxK1', 'Wilms3', ...
Features
'donor_id' = 'Wilms3', 'TTx', 'pRCC', 'VHL', 'RCC3', 'TxK1', 'TxK4', 'TxK3', 'RCC2', 'Wilms2', ...
'organism' = 'human'
'suspension_type' = 'cell'
Feature sets
'obs' = 'assay', 'cell_type', 'development_stage', 'disease', 'donor_id', 'self_reported_ethnicity', 'sex', 'tissue', 'organism', 'tissue_type', 'suspension_type'
'var' = 'None', 'EBF1', 'LINC02202', 'RNF145', 'LINC01932', 'UBLCP1', 'IL12B', 'LINC01845', 'LINC01847', 'ADRA1B', 'TTC1', 'PWWP2A', 'FABP6', 'FABP6-AS1', 'CCNJL', 'C1QTNF2'
More ways of accessing metadata
Access just features:
artifact.features
Or get labels given a feature:
artifact.labels.get(features.tissue).df()
artifact.labels.get(features.collection).one()
If you want to query a slice of the array data, you have two options:
Cache & load the entire array into memory via
artifact.load() -> AnnData
(caches the h5ad on disk, so that you only download once)Stream the array from the cloud using a cloud-backed accessor
artifact.open() -> AnnDataAccessor
Both options will run much faster if you run them close to the data (AWS S3 on the US West Coast, consider logging into hosted compute there).
Cache & load:
adata = artifact.load()
adata
Show code cell output
AnnData object with n_obs × n_vars = 7803 × 32839
obs: 'donor_id', 'donor_age', 'self_reported_ethnicity_ontology_term_id', 'organism_ontology_term_id', 'sample_uuid', 'tissue_ontology_term_id', 'development_stage_ontology_term_id', 'suspension_uuid', 'suspension_type', 'library_uuid', 'assay_ontology_term_id', 'mapped_reference_annotation', 'is_primary_data', 'cell_type_ontology_term_id', 'author_cell_type', 'disease_ontology_term_id', 'reported_diseases', 'sex_ontology_term_id', 'compartment', 'Experiment', 'Project', 'tissue_type', 'cell_type', 'assay', 'disease', 'organism', 'sex', 'tissue', 'self_reported_ethnicity', 'development_stage', 'observation_joinid'
var: 'feature_is_filtered', 'feature_name', 'feature_reference', 'feature_biotype', 'feature_length'
uns: 'citation', 'default_embedding', 'schema_reference', 'schema_version', 'title'
obsm: 'X_umap'
Now we have an AnnData
object, which stores observation annotations matching our artifact-level query in the .obs
slot, and we can re-use almost the same query on the array-level.
See the array-level query
adata_slice = adata[
adata.obs.cell_type.isin(
[cell_types.dendritic_cell.name, cell_types.neutrophil.name]
)
& (adata.obs.tissue == tissues.kidney.name)
& (adata.obs.suspension_type == suspension_types.cell.name)
& (adata.obs.assay == experimental_factors.ln_10x_3_v2.name)
]
adata_slice
See the artifact-level query for comparison
query = collection.artifacts.filter(
organism=organisms.human,
cell_types__in=[cell_types.dendritic_cell, cell_types.neutrophil],
tissues=tissues.kidney,
ulabels=suspension_types.cell,
experimental_factors=experimental_factors.ln_10x_3_v2,
)
AnnData
uses pandas to manage metadata and the syntax differs slightly. However, the same metadata records are used.
Stream:
adata_backed = artifact.open()
adata_backed
Show code cell output
AnnDataAccessor object with n_obs × n_vars = 7803 × 32839
constructed for the AnnData object 20d87640-4be8-487f-93d4-dce38378d00f.h5ad
obs: ['Experiment', 'Project', '_index', 'assay', 'assay_ontology_term_id', 'author_cell_type', 'cell_type', 'cell_type_ontology_term_id', 'compartment', 'development_stage', 'development_stage_ontology_term_id', 'disease', 'disease_ontology_term_id', 'donor_age', 'donor_id', 'is_primary_data', 'library_uuid', 'mapped_reference_annotation', 'observation_joinid', 'organism', 'organism_ontology_term_id', 'reported_diseases', 'sample_uuid', 'self_reported_ethnicity', 'self_reported_ethnicity_ontology_term_id', 'sex', 'sex_ontology_term_id', 'suspension_type', 'suspension_uuid', 'tissue', 'tissue_ontology_term_id', 'tissue_type']
obsm: ['X_umap']
raw: ['X', 'var', 'varm']
uns: ['citation', 'default_embedding', 'schema_reference', 'schema_version', 'title']
var: ['_index', 'feature_biotype', 'feature_is_filtered', 'feature_length', 'feature_name', 'feature_reference']
We now have an AnnDataAccessor
object, which behaves much like an AnnData
, and the query looks the same.
See the query
adata_backed_slice = adata_backed[
adata_backed.obs.cell_type.isin(
[cell_types.dendritic_cell.name, cell_types.neutrophil.name]
)
& (adata_backed.obs.tissue == tissues.kidney.name)
& (adata_backed.obs.suspension_type == suspension_types.cell.name)
& (adata_backed.obs.assay == experimental_factors.ln_10x_3_v2.name)
]
adata_backed_slice.to_memory()
Train ML models¶
You can directly train ML models on very large collections of AnnData objects.
Exploring data by collection¶
Alternatively,
you can search a file on the LaminHub UI and fetch it through:
ln.Artifact.get(uid)
or query for a collection you found on CZ CELLxGENE Discover
Let’s search the collections from CELLxGENE within the 2023-12-15 release:
ln.Collection.filter(version="2024-07-01").search("immune human kidney", limit=10)
<QuerySet [Collection(uid='PWDH0VJMkhsYyHwgIhN9', version='2024-07-01', name='A cell atlas of human thymic development defines T cell repertoire formation', description='10.1126/science.aay3224', hash='kdNuiUsjslVtg4wPapRn', reference='de13e3e2-23b6-40ed-a413-e9e12d7d3910', reference_type='CELLxGENE Collection ID', visibility=1, created_by_id=1, transform_id=22, run_id=27, updated_at='2024-07-16 12:24:38 UTC'), Collection(uid='YVo6IaHRKZfDxJLMfiP8', version='2024-07-01', name='Spatial and cell type transcriptional landscape of human cerebellar development', description='10.1038/s41593-021-00872-y', hash='n-9_rjprIyWeU9SFPEtL', reference='1b014f39-f202-45ae-bb7d-9286bddd8d8b', reference_type='CELLxGENE Collection ID', visibility=1, created_by_id=1, transform_id=22, run_id=27, updated_at='2024-07-16 12:24:38 UTC'), Collection(uid='cwFRDKcBVLQ1DgA4O6nC', version='2024-07-01', name='Single cell transcriptomic profiling identifies molecular phenotypes of newborn human lung cells', description='10.3390/genes15030298', hash='P4dNll_9XIdx7s4kAugC', reference='28e9d721-6816-48a2-8d0b-43bf0b0c0ebc', reference_type='CELLxGENE Collection ID', visibility=1, created_by_id=1, transform_id=22, run_id=27, updated_at='2024-07-16 12:17:42 UTC'), Collection(uid='vUL4bLnfnvI2hRpBSfK5', version='2024-07-01', name='Single-cell transcriptomics of human T cells reveals tissue and activation signatures in health and disease', description='10.1038/s41467-019-12464-3', hash='m4ds_3ZpriUHatzF7H97', reference='24d42e5e-ce6d-45ff-a66b-a3b3b715deaf', reference_type='CELLxGENE Collection ID', visibility=1, created_by_id=1, transform_id=22, run_id=27, updated_at='2024-07-16 12:24:38 UTC'), Collection(uid='lPs6VN8t49wQK3pl71dM', version='2024-07-01', name='A single-cell and spatially resolved atlas of human breast cancers', description='10.1038/s41588-021-00911-1', hash='KfY95LtPo2L8RqP612ug', reference='dea97145-f712-431c-a223-6b5f565f362a', reference_type='CELLxGENE Collection ID', visibility=1, created_by_id=1, transform_id=22, run_id=27, updated_at='2024-07-16 12:18:56 UTC'), Collection(uid='uarP82A6F0cOH8dKjpQL', version='2024-07-01', name='Comparative transcriptomics reveals human-specific cortical features', description='10.1126/science.ade9516', hash='6aAYLBJvC-dOgnZxg7sd', reference='4dca242c-d302-4dba-a68f-4c61e7bad553', reference_type='CELLxGENE Collection ID', visibility=1, created_by_id=1, transform_id=22, run_id=27, updated_at='2024-07-16 12:24:38 UTC'), Collection(uid='WpJDkF942c2mHNbJ3En3', version='2024-07-01', name='Cross-tissue immune cell analysis reveals tissue-specific features in humans', description='10.1126/science.abl5197', hash='dPITKafh7mmYOfQqQyWq', reference='62ef75e4-cbea-454e-a0ce-998ec40223d3', reference_type='CELLxGENE Collection ID', visibility=1, created_by_id=1, transform_id=22, run_id=27, updated_at='2024-07-16 12:24:39 UTC'), Collection(uid='rbcRjHfXE0LKIvZcjZro', version='2024-07-01', name='Single cell RNA sequencing of human liver reveals distinct intrahepatic macrophage populations', description='10.1038/s41467-018-06318-7', hash='9f-ccLWu6VqrkN4ITb-Z', reference='bd5230f4-cd76-4d35-9ee5-89b3e7475659', reference_type='CELLxGENE Collection ID', visibility=1, created_by_id=1, transform_id=22, run_id=27, updated_at='2024-07-16 12:24:38 UTC'), Collection(uid='2gBKIwx8AtCHc4nfcQqc', version='2024-07-01', name='A single-cell transcriptome atlas of the adult human retina', description='10.15252/embj.2018100811', hash='sCh4gUTJJJjECsp1dj0q', reference='3472f32d-4a33-48e2-aad5-666d4631bf4c', reference_type='CELLxGENE Collection ID', visibility=1, created_by_id=1, transform_id=22, run_id=27, updated_at='2024-07-16 12:24:39 UTC'), Collection(uid='zZLyhpo1aDdxdbULFbVT', version='2024-07-01', name='Single-cell transcriptomic atlas of the human retina identifies cell types associated with age-related macular degeneration', description='10.1038/s41467-019-12780-8', hash='1B0m9_FahAvefSTM8_AV', reference='1a486c4c-c115-4721-8c9f-f9f096e10857', reference_type='CELLxGENE Collection ID', visibility=1, created_by_id=1, transform_id=22, run_id=27, updated_at='2024-07-16 12:24:38 UTC')]>
Let’s get the record of the top hit collection:
collection = ln.Collection.get("kqiPjpzpK9H9rdtnV67f")
collection
Collection(uid='kqiPjpzpK9H9rdtnV67f', version='2023-12-15', name='Spatiotemporal immune zonation of the human kidney', description='10.1126/science.aat5031', hash='4wGcXeeqsjVdbRdU7ZuJ', reference='120e86b4-1195-48c5-845b-b98054105eec', reference_type='CELLxGENE Collection ID', visibility=1, created_by_id=1, transform_id=17, run_id=22, updated_at='2024-01-29 07:54:33 UTC')
We see it’s a Science paper and we could find more information using the DOI or CELLxGENE collection id.
Check different versions of this collection:
collection.versions.df()
uid | version | name | description | hash | reference | reference_type | visibility | transform_id | artifact_id | run_id | created_by_id | updated_at | |
---|---|---|---|---|---|---|---|---|---|---|---|---|---|
id | |||||||||||||
17 | kqiPjpzpK9H9rdtnHWas | 2023-07-25 | Spatiotemporal immune zonation of the human ki... | 10.1126/science.aat5031 | w_VZE7n841ktaA9FjdLh | 120e86b4-1195-48c5-845b-b98054105eec | CELLxGENE Collection ID | 1 | NaN | None | NaN | 1 | 2024-01-08 12:01:20.121095+00:00 |
365 | kqiPjpzpK9H9rdtnV67f | 2023-12-15 | Spatiotemporal immune zonation of the human ki... | 10.1126/science.aat5031 | 4wGcXeeqsjVdbRdU7ZuJ | 120e86b4-1195-48c5-845b-b98054105eec | CELLxGENE Collection ID | 1 | 17.0 | None | 22.0 | 1 | 2024-01-29 07:54:33.854515+00:00 |
595 | kqiPjpzpK9H9rdtnCt1o | 2024-07-01 | Spatiotemporal immune zonation of the human ki... | 10.1126/science.aat5031 | I6mGKs5YVdoOJwMdRfj_ | 120e86b4-1195-48c5-845b-b98054105eec | CELLxGENE Collection ID | 1 | 22.0 | None | 27.0 | 1 | 2024-07-16 12:24:39.167691+00:00 |
Each collection has at least one Artifact
file associated to it. Let’s get the associated artifacts:
collection.artifacts.df()
uid | version | description | key | suffix | type | accessor | size | hash | hash_type | n_objects | n_observations | visibility | key_is_virtual | storage_id | transform_id | run_id | created_by_id | updated_at | |
---|---|---|---|---|---|---|---|---|---|---|---|---|---|---|---|---|---|---|---|
id | |||||||||||||||||||
1778 | b2x19Eg28GGSNnXW1hAD | 2023-12-15 | Fetal kidney dataset: nephron | cell-census/2023-12-15/h5ads/08073b32-d389-41f... | .h5ad | None | AnnData | 159545411 | _JE59jFHDrOn0hj4i1yXSQ-20 | md5-n | None | 10790 | 1 | False | 2 | 16 | 22 | 1 | 2024-01-29 07:46:06.497662+00:00 |
1880 | WwmBIhBNLTlRcSoBpatT | 2023-12-15 | Mature kidney dataset: immune | cell-census/2023-12-15/h5ads/20d87640-4be8-487... | .h5ad | None | AnnData | 44647761 | hSLF-GPhLXaC2tVIOJEdXA-6 | md5-n | None | 7803 | 1 | False | 2 | 16 | 22 | 1 | 2024-01-29 07:46:33.152678+00:00 |
1930 | gHlQ5Muwu3G9pvFC7egT | 2023-12-15 | Fetal kidney dataset: immune | cell-census/2023-12-15/h5ads/2d31c0ca-0233-41c... | .h5ad | None | AnnData | 64056560 | jENeQIq0JdoHl5PyfY-sjA-8 | md5-n | None | 6847 | 1 | False | 2 | 16 | 22 | 1 | 2024-01-29 07:46:37.205210+00:00 |
1944 | USUgRVwrCMquHiImhk5D | 2023-12-15 | Mature kidney dataset: non PT parenchyma | cell-census/2023-12-15/h5ads/2fc9c59f-3cfd-48d... | .h5ad | None | AnnData | 39294782 | 3l5iNnBmPFbYfR3-THYWNQ-5 | md5-n | None | 4620 | 1 | False | 2 | 16 | 22 | 1 | 2024-01-29 07:46:52.173865+00:00 |
2405 | P4Oai3OLGAzRwoicaxCB | 2023-12-15 | Mature kidney dataset: full | cell-census/2023-12-15/h5ads/9ea768a2-87ab-46b... | .h5ad | None | AnnData | 192484358 | yghldeu2bOC5jtvnqZH8Og-23 | md5-n | None | 40268 | 1 | False | 2 | 16 | 22 | 1 | 2024-01-29 07:49:11.905786+00:00 |
2570 | 6mnZ3SeQFhffr3wTdZZb | 2023-12-15 | Fetal kidney dataset: stroma | cell-census/2023-12-15/h5ads/c52de62a-058d-4d7... | .h5ad | None | AnnData | 109942751 | s24Q5-FNUNQPLZw9BuwOVg-14 | md5-n | None | 8345 | 1 | False | 2 | 16 | 22 | 1 | 2024-01-29 07:50:01.866851+00:00 |
2652 | 11HQaMeIUaOwyHoOWVvA | 2023-12-15 | Fetal kidney dataset: full | cell-census/2023-12-15/h5ads/d7dcfd8f-2ee7-438... | .h5ad | None | AnnData | 341214674 | 2mnG5TiEpj0Wr5L19TTFRw-41 | md5-n | None | 27197 | 1 | False | 2 | 16 | 22 | 1 | 2024-01-29 07:50:28.610568+00:00 |